
Research says when businesses have access to their customers' digital footprints, they can learn invaluable knowledge about their preferences, needs, and behavior. The same applies to the debt collection industry.
Success in debt collection hinges on more than persistence; it's about precision, data, strategy, and informed decision-making. This is where collections analytics becomes a game-changer. By harnessing the power of data, agencies can move from a one-size-fits-all approach to a tailored strategy that increases recovery rates and minimizes risk.
Collections analytics provides the insights needed to optimize every aspect of the collections process. It identifies high-risk accounts and predicts the best time to reach a debtor. In this article, you’ll explore how analytics revolutionizes debt collection, enhances performance at every stage, and ultimately drives more successful outcomes for agencies. Let’s start.
Definition and Role of Collections Analytics
Collections analytics involves using data and tools to manage unpaid accounts better and improve the collections process.
It analyses payment histories, customer details, credit scores, and overdue patterns to assess unpaid accounts, enabling agencies to:

- Spot High-risk Accounts: Collections analytics identifies customers likely to delay payments by analyzing overdue amounts, payment frequency, and financial condition. This helps agencies prioritize these accounts and take early action to prevent losses.
- Group Customers Better: Collections analytics helps divide customers into groups based on their payment history or risk level. For example, customers who often pay late can receive different reminders or payment plans than those who usually pay on time.
- Predict Cash Flow: By studying past data, collections analytics can predict how much money a business will likely receive in the future. This helps agencies manage their cash better, ensuring they have enough for smooth running and growth.
- Improve Collection Strategies: Detailed data allows collectors to decide when to send reminders, adjust follow-up schedules, and know when to take decisive collection steps. This data-driven approach helps reduce wasted effort and focus on accounts most likely to pay.
Poorly managed accounts receivable result in bad debt and can eventually lead to write-offs, hurting your profits. Collections analytics helps collectors spot high-risk accounts early by enabling them to adjust payment terms or offer discounts for early payments.
In addition, collections analytics helps agencies build better customer relationships by personalizing the process.
Key Benefits of Collections Analytics
Collection analytics offers many advantages by providing data-driven insights for smarter decisions and strategic actions. Here are some key benefits:

- Improved Efficiency in Debt Recovery: Collection analytics helps agencies develop targeted and personalized strategies for different customer groups. Using data, they can prioritize high-risk accounts, focus their collection efforts, and allocate resources more effectively.
- Reduced Bad Debt: Research says that risk analysis provides the foresight that enables businesses to implement preventative measures and avoid potential pitfalls. Collections analytics analyze past payment data and customer behavior to predict future payment trends. This allows agencies to manage collections proactively and reduce the chances of bad debt.
- Enhanced Cash Flow Management: Collections analytics help agencies better understand payment cycles, average Days Sales Outstanding (DSO), and other critical metrics. This insight helps optimize cash flow, improve working capital, and lower the risk of financial issues.
- Streamlined Operations: Collections analytics automates data gathering and analysis, using real-time dashboards to simplify reporting, reduce errors, and improve operational efficiency.
Stages of Collections Enhanced by Analytics
The collections process can be challenging, but using analytics at every stage helps improve results, speed up recovery, and reduce bad debt. Here's how analytics enhances collections across three key stages:
1. Early Stage: Identification of High-risk Customers and Self-cure Patterns
The early stage of collections is critical as it lays the foundation for the success of the entire recovery process. Analytics at this stage helps agencies identify high-risk customers who will likely miss payments.
Agencies can spot trends by analyzing past data, payment patterns, and customer behavior and proactively address potential issues. Here’s how analytics help in the early stage:
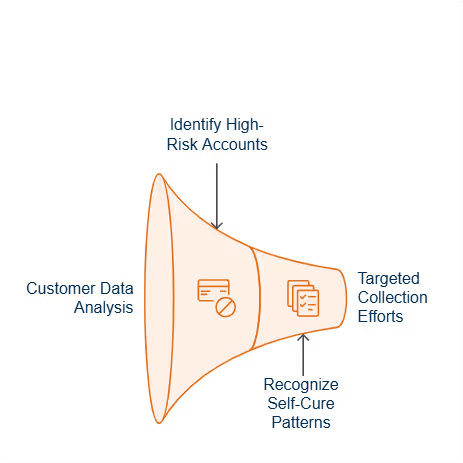
- Identification of High-Risk Accounts: Analytics helps assess payment history, credit scores, and industry-specific risk factors to classify accounts based on default risk. This allows agencies to focus on high-risk customers who need immediate attention while avoiding unnecessary follow-ups with low-risk accounts.
- Understanding Self-Cure Patterns: Data analysis can identify customers who typically resolve overdue payments independently, such as paying on the 30th day or responding to the first automated reminder. Recognizing these patterns enables agencies to automate specific tasks or monitor these accounts by saving time and resources.
Agencies can streamline collections and improve early-stage recovery outcomes by prioritizing high-risk accounts and tailoring efforts based on payment behaviors.
2. Mid-Stage: Customer Segmentation and Focusing on Segmented Customer Risk
In the mid-stage of collections, when accounts are overdue but not yet severely delinquent, analytics can help agencies segment customers more effectively. By analyzing customer behavior and risk, agencies can tailor their strategies to ensure each customer receives the appropriate level of intervention. Here’s how analytics help in the mid-stage:

- Customer Segmentation: Analytics enables agencies to group customers into risk categories based on payment history, debt age, industry, and response to past collections. For instance, high-value customers with a history of late payments but long-term loyalty can form one segment. At the same time, another might include customers with smaller debts but a higher chance of default.
- Targeted Risk Management: After segmentation, agencies can customize their approach for each group. High-risk accounts may need proactive measures like phone calls or tailored payment plans, while low-risk customers can be managed with softer tactics like email reminders. This targeted approach ensures efficient resource allocation and avoids unnecessary contact with low-risk customers.
By leveraging segmentation, agencies can make their mid-stage collections more personalized, effective, and cost-efficient.
3. Final Stage: Evaluation for Write-offs and Hardship Plan Adjustments
The final stage of collections deals with accounts that are significantly overdue and less likely to recover without extra effort. Agencies assess, write-offs and review hardship plans for necessary adjustments. Analytics helps identify which accounts are worth pursuing and which should be written off or handled through alternative strategies.

- Evaluation for Write-Offs: Analytics helps agencies determine if the cost of pursuing overdue accounts outweighs the chances of recovery. Predictive models can identify accounts that are unlikely to pay, such as those that are significantly past due or linked to customers who have filed for bankruptcy. Writing off such accounts helps manage bad debt and ensures resources are focused on recoverable cases.
- Hardship Plan Adjustments: For accounts struggling but not yet ready for write-off, analytics can highlight customers who might benefit from revised hardship plans. These adjustments could include flexible payment terms, lower interest rates, or extended deadlines. Agencies can customize their offers by analyzing customer responses to these plans to increase repayment rates while maintaining good relationships.
This data-driven evaluation allows agencies to decide which debts to write off, which customers to assist, and which accounts to pursue. It ensures a more effective and efficient final collection stage.
Core Metrics and Indicators in Collections Analytics

Effective collections analytics depends on key metrics to measure performance, manage risk, and enhance cash flow. Below are some essential metrics used in collections analytics:
Days Sales Outstanding (DSO)
Days Sales Outstanding (DSO) is a critical metric for evaluating the efficiency of the collections process. It calculates an agency's average days to collect payments after a sale. The formula for DSO is:
DSO = (Accounts Receivable / Total Credit Sales) × Number of Days
DSO provides insight into how efficiently a company converts its receivables into cash. A high DSO suggests that the company takes longer to collect payments, which can cause cash flow challenges and raise the risk of bad debt. A low DSO indicates faster collections, improved cash flow, and reduced chances of overdue payments.
Customer Behavior
Research claims that data is what allows businesses to understand customer behavior. Collections analytics helps agencies monitor customer behavior throughout the collection process.
For instance, some customers may pay late but eventually settle their debts, while others might frequently miss payments and ignore reminders. This data allows agencies to tailor their collection strategies based on each customer's payment habits.
A kind approach may work best if a customer sometimes delays payments but eventually pays everything. However, agencies might need to take stronger action, such as changing payment terms or using debt recovery services for customers with payment problems.
Agencies can also analyze data to spot signs of a customer struggling financially. In these cases, offering flexible payment plans or other help can prevent the account from defaulting.
Payment Trends
Payment trends show patterns, such as when customers are more likely to pay during the month or when specific customers regularly pay late. Some customers might make partial payments or miss due dates by a week. By spotting these patterns early, agencies can change how they handle collections, such as sending reminders sooner or setting up more organized payment plans.
Analytics can help spot customers at a higher risk of not paying. If a customer who usually pays on time starts delaying or paying less, it could be a sign of financial trouble or a payment dispute.
Recognizing these signs early allows agencies to take action, such as sending reminders more often or offering to change payment terms. This prevents accounts from becoming overdue or being written off.
Aging of Receivables
Aging receivables is the process of grouping unpaid invoices based on their length of overdue. These are usually categorized into 30, 60, 90, and 120 days past due.
Having many receivables that are 90+ days overdue can indicate problems with collections or credit policies, which, if not addressed, may lead to more bad debt.
On the other hand, a low percentage of aged receivables shows that the collections process is working well and cash flow is being managed effectively. Reviewing aging reports helps spot patterns or recurring issues with specific customers or industries.
Build a Stellar Team for Collecting Payments Digitally
Discover the roles, skills, and responsibilities you need in the team that drives significant revenue from digital channels. Read now.
Optimizing Collections Strategies with Analytics
In the evolving collections world, analytics is crucial for improving strategies by offering data-driven insights into customer behaviors, preferences, and payment patterns. Here are key ways to use analytics to enhance collection strategies:
Personalized Collection Strategies Based on Customer Data

Research says that 71% of customers expect personalized interactions with brands, and 76% express frustration when these expectations aren’t met. Personalized strategies based on customer data significantly increase the chances of getting timely payments while maintaining good customer relationships.
Have a look at how analytics helps with personalization:
- Payment History: Collectors examine past payment habits to identify customers who are often late and those who usually pay on time.
- Communication Preferences: Analytics shows how customers like to be contacted (email, phone, text, etc.). Some customers prefer email reminders over phone calls, while others may respond better to personal calls.
- Customer Demographics: Different customer groups may have specific payment behaviors based on industry, size, or financial health. Analytics helps agencies group customers by these factors and allows them to create strategies for them.
Determining Optimal Contact Methods and Times
The method and timing of contact can significantly affect the success of collections. Analytics helps agencies determine the best ways and times to reach different customers. It improves the chances of successful communication and payment.
- Optimal Contact Methods: Agencies can identify which communication channels work best for each customer by analyzing customer data. Some may respond better to automated email reminders, while others prefer a phone call or a text message. Analytics helps segment customers based on their preferences by ensuring the right contact method is used to increase response rates.
- Best Time to Contact: Analytics can also help determine the best time to contact customers for maximum effectiveness. For example, data might show that customers are more likely to pay if contacted on Monday mornings or respond faster after reminders at certain times. Agencies can adjust their contact schedules to achieve better results by understanding when customers are most responsive.
- Frequency of Contact: Analytics helps agencies find the ideal frequency for reaching customers. Too few reminders may lead to late payments, while too many could annoy customers. By finding the right balance, agencies can maintain consistent collection efforts without overwhelming their customers.
Creating Effective Contact Scripts for Enhanced Interactions
The way you communicate during the collections process can significantly affect whether you get paid. By using analytics, agencies can create better scripts for contacting customers, improving interactions, and boosting the chances of successful collections.
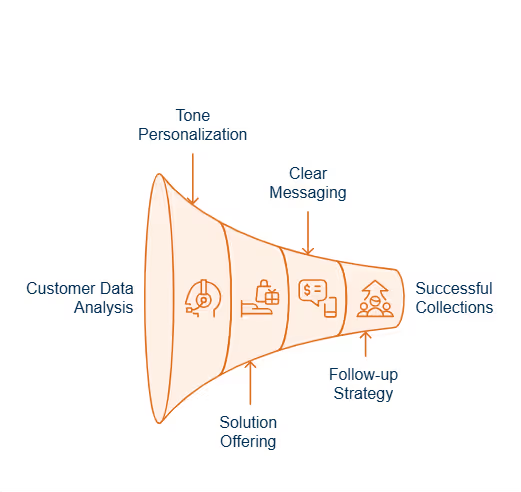
- Tone and Personalization: Analytics can help agencies understand the best tone to use when communicating with customers based on their behavior. For example, customers who are likely to pay late may need a more formal and urgent tone. On the other hand, customers who have a good payment history may respond better to a friendly and polite tone.
- Offer Solutions: Analytics can help agencies offer alternatives to struggling customers, such as payment plans, early payment discounts, or temporary suspensions of penalties. Tailoring the script to fit the customer’s financial situation can improve the chances of payment and prevent the account from being lost.
- Clear and Concise Messaging: The contact script should be straightforward. Analytics can show which wording and phrasing work best and help agencies avoid confusion. For example, scripts can highlight deadlines, provide clear payment instructions, or explain the consequences of non-payment so customers know exactly what to do.
- Follow-up Strategy: Analytics can help determine the best follow-up approach after the first contact. Should the message remind the customer of the due date or offer a payment plan option if they can’t pay everything simultaneously? Agencies can improve their follow-up strategy by testing and refining scripts based on customer responses.
Predictive Modeling in Collections Analytics
Predictive modeling is changing how collection strategies work. It uses past data and advanced analytics to predict customer behavior and help agencies make better decisions. In collections, these models allow agencies to predict when customers will likely pay, whether they might default, and the best way to recover payments.
Here’s a look at how predictive modeling works in collections, including how it is created, the key factors involved, and strategies for proactively managing payments.
Creation of Predictive Models to Forecast Customer Payment Behaviour
Predictive modeling uses past data to create models that predict future customer behavior. These models analyze large amounts of data, spotting patterns and connections that show how likely customers are to pay on time or default.
Here’s the process to create models that forecast payment behavior with greater accuracy:
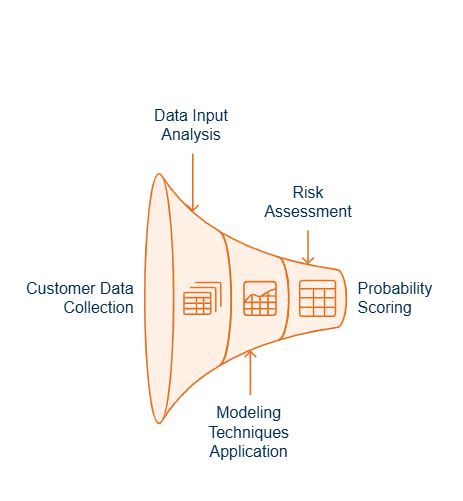
- Data Inputs: Predictive models use various data points to predict customer payment behavior. This data often includes payment history, credit scores, industry data, demographic information, and external factors like economic conditions or seasonal trends.
- Modeling Techniques: Agencies use different techniques to make predictions after collecting the data. For example, machine learning algorithms analyze past payment behaviors to identify customers at higher risk of late payments. The model then gives each customer a probability score, showing how likely they are to pay on time.
Factors Considered in Predicting Timely Payments
Whether a customer will pay on time involves examining internal and external factors. These factors help agencies better understand customer behavior and create more effective payment collection strategies.
- Payment History: A customer's past behavior is key to predicting future payments. Customers who consistently pay on time are likelier to continue doing so. On the other hand, those who often delay payments or don’t pay in full may be considered high-risk and need more attention.
- Credit Scores: A customer’s credit score is an important indicator of payment reliability. Higher credit scores usually mean better payment behavior, while lower credit scores may suggest financial problems, increasing the likelihood of delays or missed payments.
- Account Age and Invoice Frequency: The age of the account and how often invoices are sent can affect payment behavior. Older or larger invoices with longer payment terms might have more delays, while accounts with frequent, smaller invoices tend to have more consistent payments. This helps agencies focus on certain types of accounts.
- Economic and Industry Conditions: External factors, such as economic changes or issues in the customer's industry, can impact their ability to pay on time. For example, if the customer’s industry is struggling or facing supply chain issues, they may have delays in making payments.
- Engagement with Previous Collections Efforts: Customers' past responses to collections efforts, such as reminders or payment plans, can predict their behavior. Customers who respond to reminders and stick to payment plans are likelier to make payments on time later.
Strategies for Proactive Collection Management
Once predictive models are in place, agencies can use the insights to take action before payments are missed, helping to improve cash flow. Here are some strategies that predictive analytics can make possible:
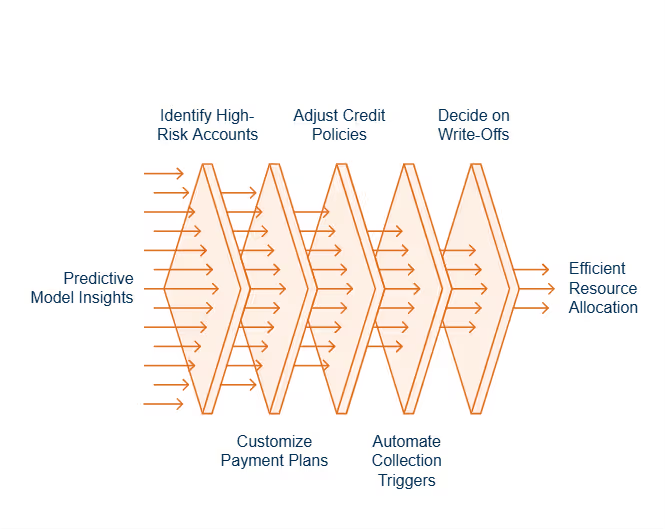
- Targeting High-Risk Accounts Early: If a predictive model predicts a customer will delay payment, the agency can reach out early with a reminder or a personalized message.
- Customized Payment Plans: Forbes claims that personalizing payment methods can help propel a business to the next level. Predictive models create tailored payment plans for high-risk customers who can still afford them.
- Dynamic Credit Management: Debt Collectors can adjust their credit policies by tracking predictive scores.
- Automated Collection Triggers: When a high-risk customer is identified, an automated system sends a reminder email, makes a phone call, or escalates the case to the collections team.
- Identifying Potential Write-Offs: If a model predicts a customer won’t pay, agencies can more confidently decide whether to write off the debt or continue further collection efforts.
- Improved Resource Allocation: Instead of spending time and effort on all accounts equally, collectors can focus on high-risk accounts that need attention.
Challenges and Overcoming Barriers in Collections Analytics

While collections analytics greatly improves the recovery process, it also presents challenges that agencies must manage. However, the right strategies can effectively handle these challenges to ensure that analytics provides real and meaningful results.
Conclusion
Collections analytics is a powerful tool that fundamentally transforms how agencies approach debt recovery. Agencies leverage data and gain deeper insights into debtor behavior, streamline their collections process, and make more informed decisions. The data helps at every stage, whether identifying high-risk accounts, optimizing communication strategies, or predicting the likelihood of successful recovery.
From core metrics to predictive modeling, analytics enables agencies to fine-tune their approaches and adapt to evolving challenges, which drives better outcomes. While implementing collections analytics can be challenging, investing in technology and talent leads to long-term success.
As debt collection becomes increasingly data-driven, those who harness the full potential of collections analytics will improve their recovery rates & build a more customer-friendly approach.
5 ways to maximize return on bill pay campaigns. Check now.
FAQs (Frequently Asked Questions)
1. What are the five methods of data collection?
The five methods of data collection are Document reviews, Interviews, Focus groups, Surveys, and Observation or testing.
2. Why is data collection important?
Data collection gathers important information for analysis and decision-making. It helps generate insights, track progress, solve problems, and enrich existing knowledge.
3. What is CRM in debt collection?
CRM software streamlines debt collection by centralizing customer data, automating reminders, tracking interactions, and providing insights into payment histories and balances, enhancing recovery strategies.